How to Predict Customer Sentiment in AEO
Published on 1/23/2025
•
10 min estimated read time
Here's What You Need to Do:
- Implement AI-powered sentiment analysis tools to track and predict brand perception across digital platforms.
- Create a data-driven content strategy by analyzing historical sentiment patterns and real-time feedback.
- Optimize your content for zero-click searches by understanding AI search fundamentals.
- Develop proactive response frameworks to manage sentiment shifts before they impact your brand.
The Basics of Sentiment Prediction
Why Predicting Customer Sentiment Matters for AI Search
Understanding customer sentiment isn't just nice to have - it's essential for visibility in AI-powered search.
Gen AI and search engines are getting smarter at gauging user intent and emotional resonance, making sentiment a key ranking factor.
When you can predict and respond to customer sentiment effectively, you're essentially speaking the language that modern search algorithms understand.
Let's Define Sentiment Analysis Fundamentals
Sentiment analysis is like being a digital emotion detective. It's the process of determining whether text expresses positive, negative, or neutral feelings.
Think of it as teaching computers to understand the emotional tone behind words (just like how you can tell when your friend is excited, even in a text message).
The foundation rests on natural language processing (NLP) and machine learning algorithms that scan text for emotional indicators. These systems look at:
- Word choice and context
- Sentence structure
- Emotional intensity
- Linguistic patterns
Pro tip: When choosing a sentiment analysis tool, look for one that can handle industry-specific jargon and contextual nuances - it'll give you much more accurate results.
Use AI for Accurate Sentiment Forecasting
Leverage machine learning algorithms
Machine learning algorithms have revolutionized how we predict and understand brand sentiment. These sophisticated systems can process vast amounts of unstructured data to identify patterns that human analysts might miss.
The key lies in training these algorithms with high-quality, relevant data that reflects your specific market context.
Successful implementation requires a deep understanding of both the technical capabilities and practical limitations of different ML models.
Some algorithms excel at processing text-based feedback, while others are better suited for analyzing visual content or social media interactions.
Pro tip: Focus on training your ML models with industry-specific data to improve accuracy and relevance of sentiment predictions. This targeted approach yields more actionable insights than generic sentiment analysis.
Historical sentiment data 101
Historical sentiment data serves as the foundation for accurate forecasting.
By examining past trends and patterns, marketers can identify seasonal fluctuations, recurring themes, and long-term shifts in brand perception.
This historical context is crucial for building predictive models that can anticipate future sentiment shifts.
The key to effective historical analysis lies in proper data segmentation and contextualization. Consider factors like:
- Market conditions during different periods
- Competitive landscape changes
- External events that might have influenced sentiment
- Campaign performance and its impact on overall sentiment
Natural language processing
Natural language processing (NLP) technology has become increasingly sophisticated in understanding the nuances of human communication.
Modern NLP systems can detect subtle variations in tone, context, and intent that might be missed by traditional sentiment analysis tools.
This capability is particularly valuable when analyzing performance metrics across different platforms.
Effective NLP implementation requires careful consideration of language variations, cultural contexts, and industry-specific terminology. The technology must be calibrated to understand not just the words being used, but also the broader context in which they appear.
How to Master Real-Time Sentiment Tracking Techniques
Monitor brand mentions across platforms
Real-time brand mention monitoring requires a sophisticated approach to data collection and analysis.
The key is establishing comprehensive coverage across all relevant platforms while maintaining the ability to filter and prioritize meaningful mentions.
This involves setting up robust tracking systems that can capture mentions across social media, news sites, forums, and location-specific contexts.
Effective monitoring goes beyond simple keyword tracking. It requires understanding the context of each mention, the influence of the source, and the potential impact on overall brand sentiment.
This comprehensive approach helps identify emerging trends and potential issues before they escalate.
Pro tip: Create custom alert thresholds for different types of mentions based on source authority and potential impact. This helps prioritize your response efforts effectively.
Assess sentiment in AI-generated content
As AI-generated content becomes more prevalent, understanding how it influences and reflects brand sentiment is crucial.
This requires specialized tools and approaches that can distinguish between human and AI-generated content while accurately assessing the sentiment in both.
The challenge lies in understanding how AI systems interpret and present information about your brand. This includes:
- Analyzing AI-generated summaries and recommendations
- Monitoring automated content aggregators
- Tracking sentiment in AI-powered customer service interactions
- Evaluating AI-generated product reviews and comparisons
Identify sentiment trends in real-time
Real-time trend identification requires both technological capability and human insight. The key is establishing systems that can process large volumes of data quickly while maintaining accuracy in sentiment assessment.
This involves using advanced analytics tools that can identify emerging patterns and potential sentiment shifts as they occur.
Successful trend identification relies on understanding the difference between normal sentiment fluctuations and significant shifts that require attention. This requires establishing baseline metrics and defining clear thresholds for what constitutes a meaningful trend.
Optimize Content for Positive Sentiment
Craft emotion-evoking brand messages
Creating content that resonates emotionally with your audience requires a deep understanding of psychological triggers and emotional marketing principles.
The most effective brand messages combine authentic storytelling with strategic emotional appeals that align with your brand values and audience expectations.
Successful emotional content follows a clear framework:
- Identify core emotional triggers for your target audience
- Develop authentic narratives that connect with these emotions
- Test and refine messaging based on sentiment feedback
- Scale successful emotional approaches across channels
Pro tip: Build a sentiment map for your most successful content pieces to identify emotional patterns that drive positive engagement. Use these insights to guide future content creation.
Tailor content to audience preferences
Personalization has become crucial in driving positive sentiment. Understanding and adapting to audience preferences requires sophisticated data analysis and content optimization strategies.
This involves analyzing engagement patterns, sentiment responses, and behavioral data to create content that truly resonates.
The key to effective content tailoring lies in understanding the subtle differences between audience segments and how they respond to different content types.
This includes considering factors like preferred content formats, tone of voice, and topic preferences.
Enhance visibility in AI answer engines
Optimizing for AI answer engines requires a new approach to content creation and optimization. This involves understanding how AI systems evaluate and present content, and adapting your strategy to ensure positive sentiment in AI-generated responses.
Success in AI answer engines depends on creating content that is both informative and emotionally resonant.
This requires balancing technical optimization with authentic, engaging storytelling that appeals to both human readers and AI systems.
How to Predict Sentiment in Zero-Click Searches
Understand AI-powered search behavior
AI-powered search behavior represents a fundamental shift in how users discover and interact with brand content. Understanding these patterns requires a deep dive into how AI systems interpret and present information in zero-click environments.
This knowledge is crucial for maintaining positive brand sentiment in an increasingly AI-driven search landscape.
The key to mastering AI search behavior lies in understanding the factors that influence AI's content selection and presentation.
This includes analyzing query patterns, context interpretation, and the way AI systems evaluate content authority and relevance.
Pro tip: Create content that directly addresses common AI-generated answers to improve your chances of being cited as a primary source. This builds authority in zero-click environments.
Optimize for generative search results
Generative search optimization requires a somewhat different approach than traditional SEO.
Success in this environment depends on creating content that AI systems can easily understand, verify, and confidently present to users. This involves structuring information in ways that facilitate accurate sentiment interpretation by AI systems.
Effective optimization strategies include:
- Developing clear, authoritative content structures
- Providing comprehensive context for key information
- Maintaining consistent brand messaging across platforms
- Creating content that anticipates and answers follow-up questions
Forecast sentiment in AI platforms
Predicting how AI platforms will interpret and present your brand requires a deep understanding of AI content evaluation mechanisms.
This involves analyzing how different AI systems process and evaluate sentiment, and how they determine which content to surface in response to user queries.
Successful sentiment forecasting in AI platforms depends on maintaining consistent brand messaging while adapting to the unique requirements of each platform. This includes understanding how different AI systems weight various content factors and how they evaluate sentiment in different contexts.
Develop a Proactive Sentiment Strategy
Anticipate potential sentiment shifts
Developing a proactive approach to sentiment management requires the ability to identify and prepare for potential shifts before they occur.
This involves combining historical data analysis with real-time monitoring to spot early warning signs of sentiment changes.
Effective anticipation strategies require:
- Regular analysis of industry trends and competitor movements
- Monitoring of social and cultural shifts that could impact brand perception
- Development of early warning systems for potential issues
- Creation of response frameworks for different scenarios
Pro tip: Build a sentiment shift prediction model based on historical triggers and patterns to anticipate potential changes before they impact your brand.
Create sentiment-driven content plans
Content planning that takes sentiment prediction into account allows for more strategic and effective communication. This approach involves creating content that not only addresses current sentiment but also helps shape future perceptions.
The key to successful sentiment-driven content planning lies in understanding the relationship between content types and sentiment outcomes. This includes analyzing how different content formats, tones, and distribution channels impact overall brand perception.
Benchmark against industry averages
Understanding your sentiment performance in relation to industry standards provides crucial context for strategy development. This involves regular comparison of your sentiment metrics against competitors and industry benchmarks to identify areas for improvement and opportunities for differentiation.
Effective benchmarking requires establishing clear metrics and measurement frameworks that allow for meaningful comparison across different brands and market segments.
Conclusion: Customer Sentiment for Generative Engine Optimization
The future of brand success lies in mastering sentiment prediction and management in AI-driven environments.
By combining sophisticated sentiment analysis tools with proactive strategy development, marketers can stay ahead of perception shifts and maintain positive brand sentiment across all platforms.
As AI continues to reshape how consumers discover and interact with brands, the ability to predict and influence sentiment becomes increasingly crucial. Ready to transform your brand's approach to sentiment prediction?
Start implementing these strategies today to secure your position in the AI-driven future of marketing.
Frequently Asked Questions
- Q: How accurate are AI-powered sentiment prediction tools?
- A: Modern AI sentiment tools achieve accuracy rates of 85-95% when properly trained with industry-specific data. However, accuracy can vary based on context and the complexity of the sentiment being analyzed.
- Q: How often should I monitor brand sentiment?
- A: Real-time monitoring is ideal, but at minimum, conduct daily sentiment analysis for key brand mentions and weekly comprehensive reviews of sentiment trends.
- Q: What's the difference between traditional SEO and AI answer engine optimization?
- A: While traditional SEO focuses on ranking in search results, AI answer engine optimization prioritizes being cited as a source in AI-generated responses and maintaining positive sentiment in zero-click environments.
- Q: How can I improve my brand's sentiment in AI-generated answers?
- A: Focus on creating authoritative, well-structured content that directly addresses common queries, maintains consistent messaging, and provides comprehensive context for AI systems to understand and cite.
- Q: What are the key metrics for measuring sentiment success?
- A: Key metrics include sentiment score, mention frequency, response rate, sentiment trend velocity, and benchmark comparisons against industry averages.
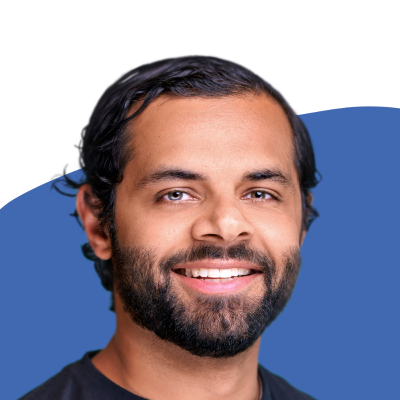
Article by
Jason Patel
Jason is an exited founder and SEO expert. He led organic growth efforts at his last company, which generated industry-leading traffic and leads with minimal outside funding. Jason is a military history dork and BJJ purple belt.